For Pycon 2025, I created a poster exploring vector embedding models, which you can download at full-size. In this post, I'll translate that poster into words.
Vector embeddings
A vector embedding is a mapping from an input (like a word, list of words, or image) into a list of floating point numbers. That list of numbers represents that input in the multidimensional embedding space of the model. We refer to the length of the list as its dimensions, so a list with 1024 numbers would have 1024 dimensions.
Embedding models
Each embedding model has its own dimension length, allowed input types, similarity space, and other characteristics.
word2vec
For a long time, word2vec was the most well-known embedding model. It could only accept single words, but it was easily trainable on any machine, it is very good at representing the semantic meaning of words. A typical word2vec model outputs vectors of 300 dimensions, though you can customize that during training. This chart shows the 300 dimensions for the word "queen" from a word2vec model that was trained on a Google News dataset:
text-embedding-ada-002
When OpenAI came out with its chat models, it also offered embedding models, like text-embedding-ada-002 which was released in 2022. That model was significant for being powerful, fast, and significantly cheaper than previous models, and is still used by many developers. The text-embedding-ada-002 model accepts up to 8192 "tokens", where a "token" is the unit of measurement for the model (typically corresponding to a word or syllable), and outputs 1536 dimensions. Here are the 1536 dimensions for the word "queen":
Notice the strange spike downward at dimension 196? I found that spike in every single vector embedding generated from the model - short ones, long ones, English ones, Spanish ones, etc. For whatever reason, this model always produces a vector with that spike. Very peculiar!
text-embedding-3-small
In 2024, OpenAI announced two new embedding models, text-embedding-3-small and text-embedding-3-large, which are once again faster and cheaper than the previous model. For this post, we'll use the text-embedding-3-small model as an example. Like the previous model, it accepts 8192 tokens, and outputs 1536 dimensions by default. As we'll see later, it optionally allows you to output less dimensions. Here are the 1536 dimensions for the word "queen":
This time, there is no downward spike, and all of the values look well distributed across the positive and negative.
Similarity spaces
Why go through all this effort to turn inputs into embeddings? Once we have embeddings of different inputs from the same embedding model, then we can compare the vectors using a distance metric, and determine the relative similarity of inputs. Each model has its own "similarity space", so the similarity rankings will vary across models (sometimes only slightly, sometimes significantly). When you're choosing a model, you want to make sure that its similarity rankings are well aligned with human rankings.
For example, let's compare the embedding for "dog" to the embeddings for 1000 common English words, across each of the models, using the cosine similarity metric.
word2vec similarity
For the word2vec model, here are the closest words to "dog", and the similarity distribution across all 1000 words:
As you can see, the cosine similarity values range from 0 to 0.76, with most values clustered between 0 and 0.2.
text-embedding-ada-002 similarity
For the text-embedding-ada-002 model, the closest words and similarity distribution is quite different:
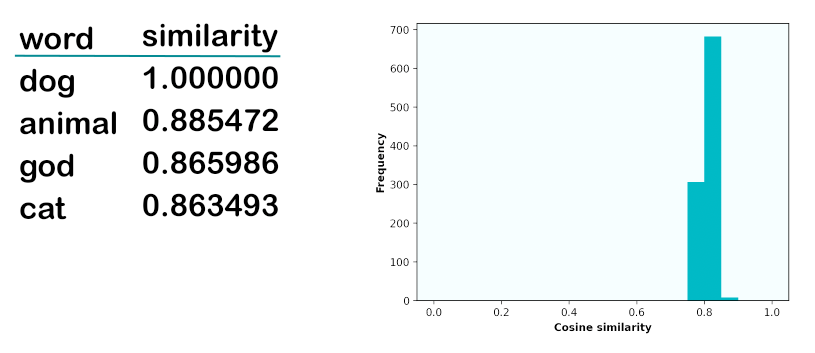
Curiously, the model thinks that "god" is very similar to "dog". My theory is that OpenAI trained this model in a way that made it pay attention to spelling similarities, since that's the main way that "dog" and "god" are similar. Another curiousity is that the similarity values are in a very tight range, between 0.75 and 0.88. Many developers find that unintuitive, as we might see a value of 0.75 initially and think it indicates a very similar value, when it actually is the opposite for this model. That's why it's so important to look at relative similarity values, not absolute. Or, if you're going to look at absolute values, you must calibrate your expectations first based on the standard similarity range of each model.
text-embedding-3-small similarity
The text-embedding-3-small model looks more similar to word2vec in terms of its closest words and similarity distribution:
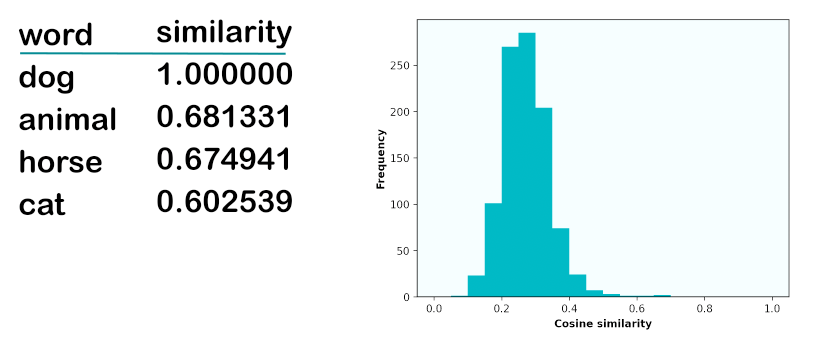
The most similar words are all similarity in semantics only, no spelling, and the similarity values peak at 0.68, with most values between 0.2 and 0.4. My theory is that OpenAI saw the weirdness in the text-embedding-ada-002 model and cleaned it up in the text-embedding-3 models.
Vector similarity metrics
There are multiple metrics we could possibly use to decide how "similar" two vectors are. We need to get a bit more math-y to understand the metrics, but I've found it helpful to know enough about metrics so that I can pick the right metric for each scenario.
Cosine similarity
The cosine similarity metric is the most well known way to measure the similarity of two vectors, by taking the cosine of the angle between the two vectors.
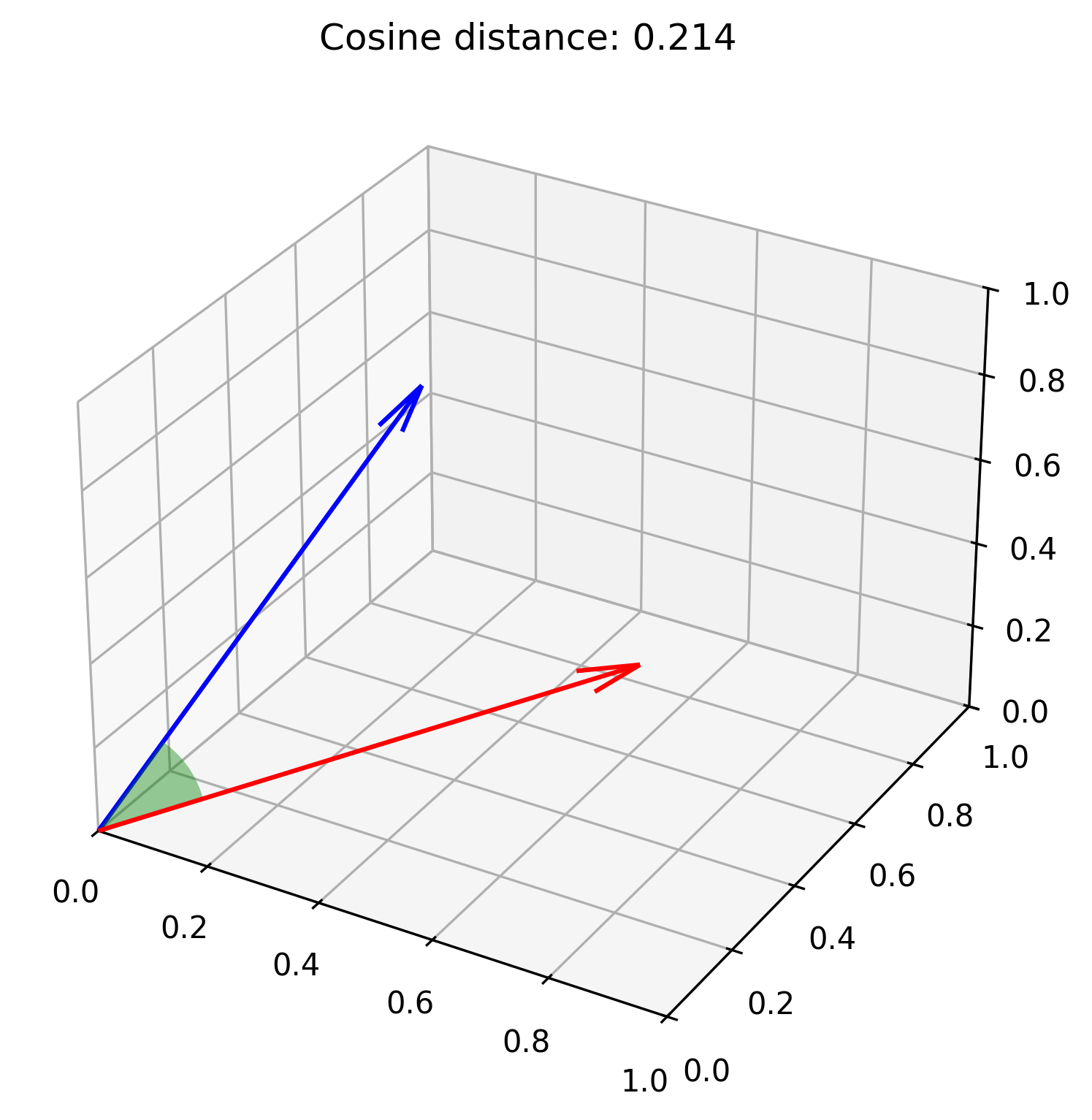
For cosine similarity, the highest value of 1.0 signifies the two vectors are the most similar possible (overlapping completely, no angle between them). The lowest theoretical value is -1.0, but as we saw earlier, modern embedding models models tend to have a more narrow angular distribution, so all the cosine similarity values end up higher than 0.
Here's the formal definition for cosine similarity:
That formula divides the dot product of the vectors by the product of their magnitudes.
Dot product
The dot product is a metric that can be used on its own to measure similarity. The dot product sums up the products of corresponding vector elements:
Here's what's interesting: cosine similarity and dot product produce the same exact values for unit vectors. How come? A unit vector has a magnitude of 1, so the product of the magnitude of two unit vectors is also 1, which means that the cosine similarity formula simplifies to the dot product formula in that special case.
Many of the popular embedding models do indeed output unit vectors, like text-embedding-ada-002 and text-embedding-3-small. For models like those, we can sometimes get performance speedups from a vector database by using the simpler dot product metric instead of cosine similarity, since the database can skip the extra step to calculate the denominator.
Vector distance metrics
Most vector databases also support distance metrics, where a smaller value indicates higher similarity. Two of them are related to the similarity metrics we just discussed: cosine distance is the complement of cosine similarity, and negative inner product is the negation of the dot product.
The Euclidean distance between two vectors is the straight-line distance between the vectors in multi-dimensional space - the path a bird would take to get straight from vector A to vector B.
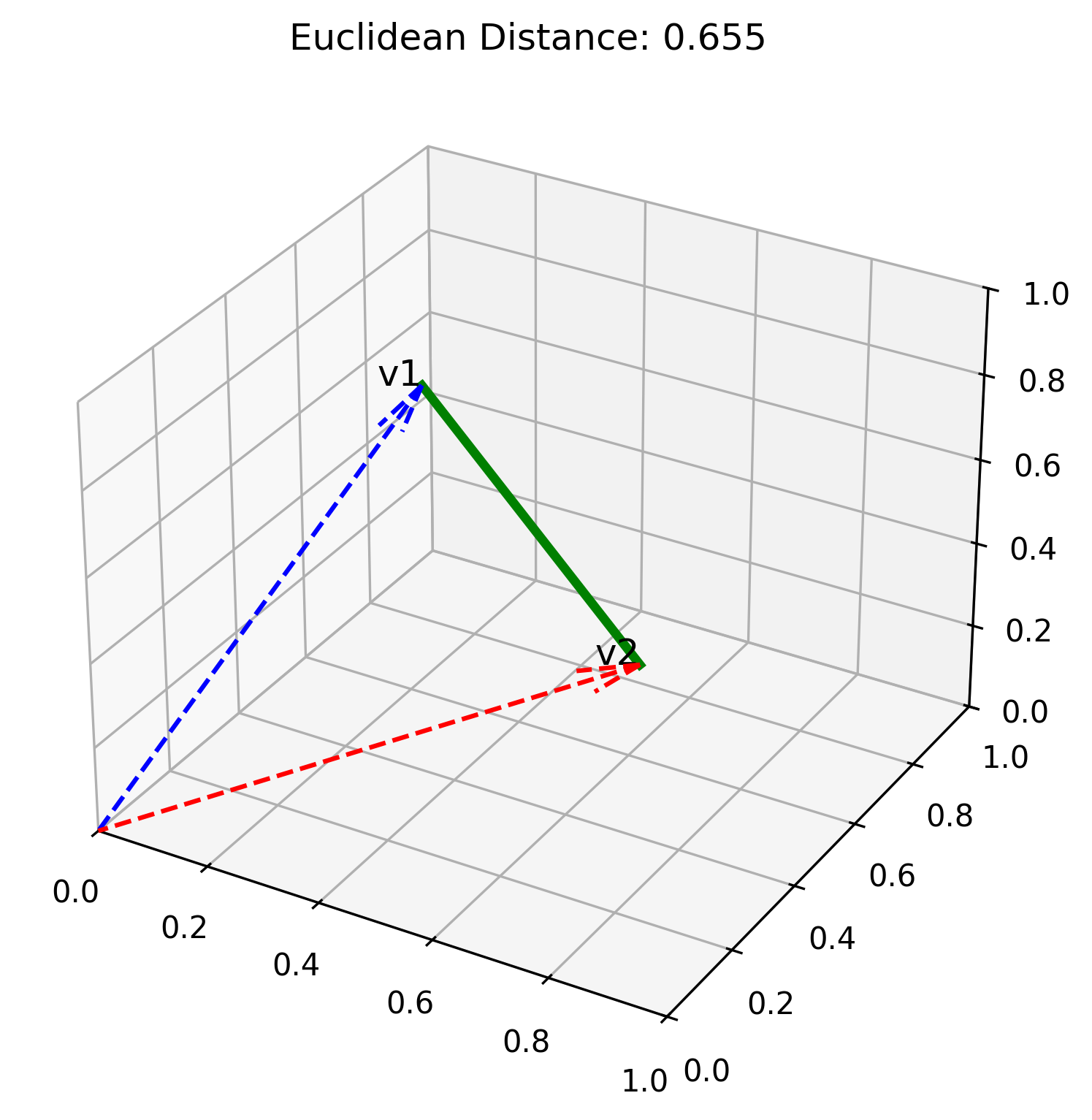
The formula for calculating Euclidean distance:
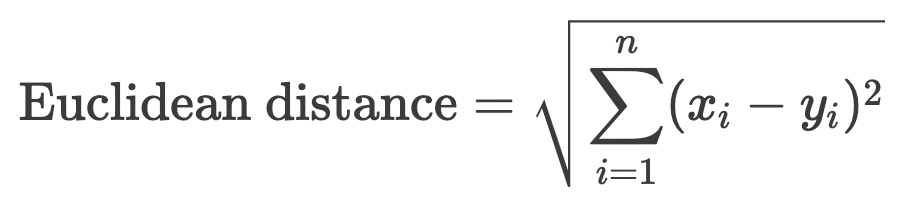
The Manhattan distance is the "taxi-cab distance" between the vectors - the path a car would need to take along each dimension of the space. This distance will be longer than Euclidean distance, since it can't take any shortcuts.
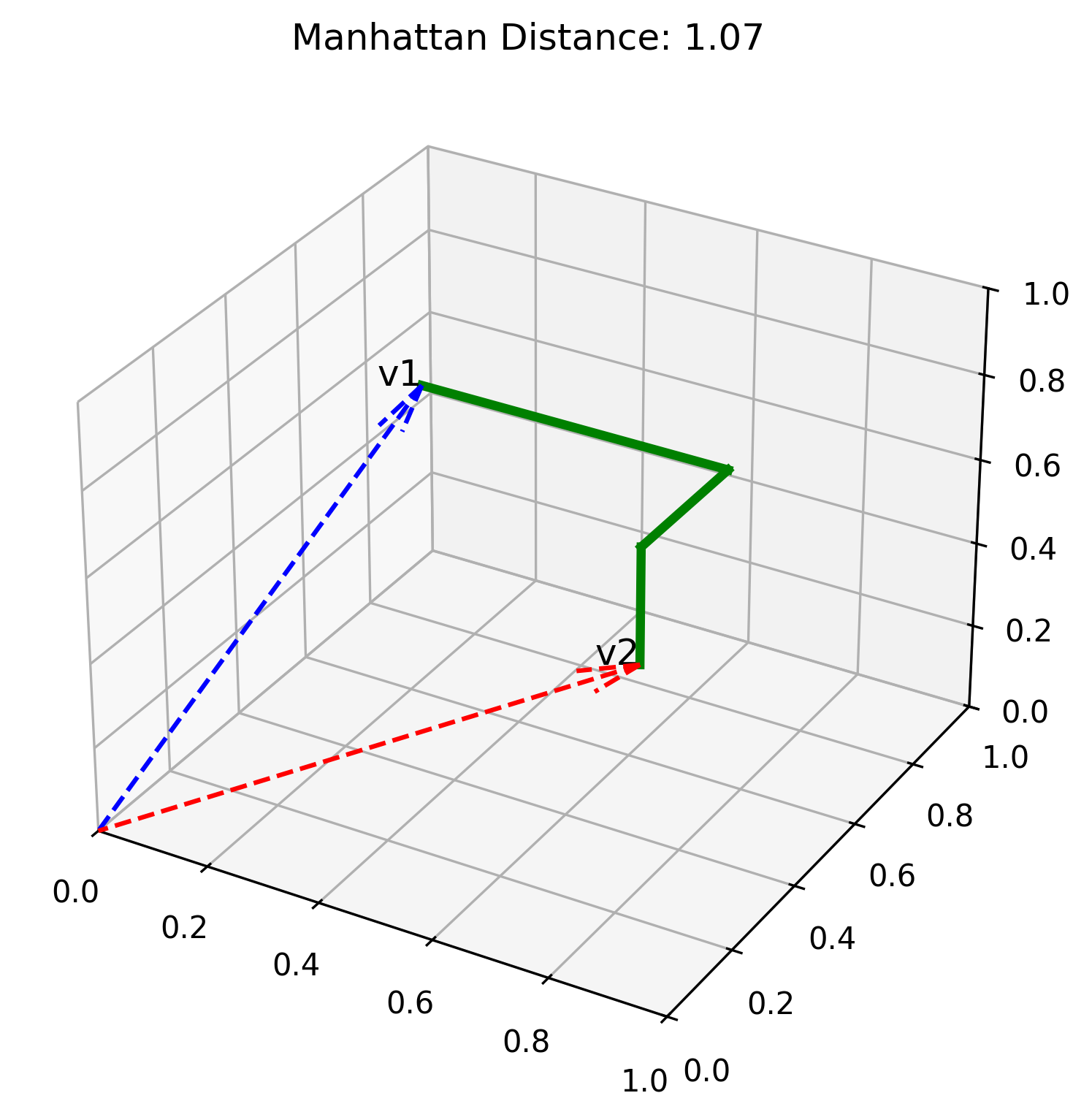
The formula for Manhattan distance:
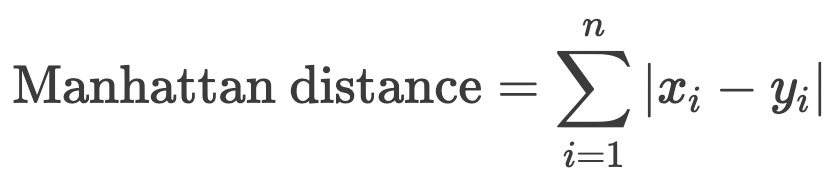
When would you use Euclidean or Manhattan? We don't typically use these metrics with text embedding models, like all the ones we've been exploring in those post. However, if you are working with a vector where each dimension has a very specific meaning and has been constructed with per-dimension meaning intentionally, then these distance metrics may be the best ones for the job.
Vector search
Once we can compute the similarity between two vectors, we can also compute the similarity between an arbitrary input vector and the existing vectors in a database. That's known as vector search, and it's the primary use case for vector embeddings these days. When we use vector search, we can find anything that is similar semantically, not just similar lexicographically. We can also use vector search across languages, since embedding models are frequently trained on more than just English data, and we can use vector search with images as well, if we use a multimodal embedding model that was trained on both text and images.

When we have a small number of vectors, we can do an exhaustive search, measuring the similarity between the input vector and every single stored vector, and returning the full ranked list.
However, once we start growing our vector database size, we typically need to use an Approximate Nearest Neighbors (ANN) algorithm to search the embedding space heuristically. A popular algorithm is HNSW, but other algorithms can also be used, depending on what your vector database supports and your application requirements.
Algorithm | Python package | Example database support |
---|---|---|
HNSW | hnswlib |
PostgreSQL pgvector extension Azure AI Search Chromadb Weaviate |
DiskANN | diskannpy | Cosmos DB |
IVFFlat | faiss | PostgreSQL pgvector extension |
Faiss | faiss | None, in-memory index only* |
Vector compression
When our database grows to include millions or even billions of vectors, we start to feel the effects of vector size. It takes a lot of space to store thousands of floating point numbers, and it takes up computation time to calculate their similarity. There are two techniques that we can use to reduce vector size: quantization and dimension reduction.
Scalar quantization
A floating point number requires significant storage space, either 32 bits or 64 bits. The process of scalar quantization turns each floating point number into an 8-bit signed integer. First, the minimum and maximum values are determined, based off either the current known values, or a hardcoded min/max for the given embedding model. Then, each floating point number is re-mapped to a number between -127 to 128.
The resulting list of integers requires ~13% of the original storage, but can still be used for similarity and search, with similar outputs. For example, compare the most similar movie titles to "Moana" between the original floating point vectors and the scalar quantized vectors:
Binary quantization
A more extreme form of compression is binary quantization: turning each floating point number into a single bit, 0 or 1. For this process, the centroid between the minimum and maximum is determined, and any lower value becomes 0 while any higher value becomes 1.
In theory, the resulting list of bits requires only 13% of the storage needed for scalar quantization, but that's only the case if the vector database supports bit-packing - if it has the ability to store multiple bits into a single byte of memory. Incredibly, the list of bits still retains a lot of the original semantic information. Here's a comparison once again for "Moana", this time between the scalar and binary quantized vectors:
Dimension reduction
Another way to compress vectors is to reduce their dimensions - to shorten the length of the list. This is only possible in models that were trained to support Matryoska Representation Learning (MRL). Fortunately, many newer models like text-embedding-3 were trained with MRL and thus support dimension reduction. In the case of text-embedding-3-small, the default/maximum dimension count is 1536, but the model can be reduced all the way down to 256.
You can reduce the dimensions for a vector either via the API call, or you can do it yourself, by slicing the vector and normalizing the result. Here's a comparison of the values between a full 1536 dimension vector and its reduced 256 version, for text-embedding-3-small:
Compression with rescoring
For optimal compression, you can combine both quantization and dimension reduction:
However, you will definitely see a quality degradation for vector search results. There's a way you can both save on storage and get high quality results, however:
- For the vector index, use the compressed vectors
- Store the original vectors as well, but don't index them
- When performing a vector search, oversample: request 10x the N that you actually need
- For each result that comes back, swap their compressed vector with original vector
- Rescore every result using the original vectors
- Only use the top N of the rescored results
That sounds like a fair bit of work to implement yourself, but databases like Azure AI Search offer rescoring as a built-in feature, so you may find that your vector database makes it easy for you.
Additional resources
If you want to keep digging into vector embeddings:
- Explore the Jupyter notebooks that generated all the visualizations above
- Check out the links at the bottom of each of those notebooks for further learning
- Watch my talk about vector embeddings from the Python + AI series