Review
Abstract
Background: Depression affects more than 350 million people globally. Traditional diagnostic methods have limitations. Analyzing textual data from social media provides new insights into predicting depression using machine learning. However, there is a lack of comprehensive reviews in this area, which necessitates further research.
Objective: This review aims to assess the effectiveness of user-generated social media texts in predicting depression and evaluate the influence of demographic, language, social media activity, and temporal features on predicting depression on social media texts through machine learning.
Methods: We searched studies from 11 databases (CINHAL [through EBSCOhost], PubMed, Scopus, Ovid MEDLINE, Embase, PubPsych, Cochrane Library, Web of Science, ProQuest, IEEE Explore, and ACM digital library) from January 2008 to August 2023. We included studies that used social media texts, machine learning, and reported area under the curve, Pearson r, and specificity and sensitivity (or data used for their calculation) to predict depression. Protocol papers and studies not written in English were excluded. We extracted study characteristics, population characteristics, outcome measures, and prediction factors from each study. A random effects model was used to extract the effect sizes with 95% CIs. Study heterogeneity was evaluated using forest plots and P values in the Cochran Q test. Moderator analysis was performed to identify the sources of heterogeneity.
Results: A total of 36 studies were included. We observed a significant overall correlation between social media texts and depression, with a large effect size (r=0.630, 95% CI 0.565-0.686). We noted the same correlation and large effect size for demographic (largest effect size; r=0.642, 95% CI 0.489-0.757), social media activity (r=0.552, 95% CI 0.418-0.663), language (r=0.545, 95% CI 0.441-0.649), and temporal features (r=0.531, 95% CI 0.320-0.693). The social media platform type (public or private; P<.001), machine learning approach (shallow or deep; P=.048), and use of outcome measures (yes or no; P<.001) were significant moderators. Sensitivity analysis revealed no change in the results, indicating result stability. The Begg-Mazumdar rank correlation (Kendall τb=0.22063; P=.058) and the Egger test (2-tailed t34=1.28696; P=.207) confirmed the absence of publication bias.
Conclusions: Social media textual content can be a useful tool for predicting depression. Demographics, language, social media activity, and temporal features should be considered to maximize the accuracy of depression prediction models. Additionally, the effects of social media platform type, machine learning approach, and use of outcome measures in depression prediction models need attention. Analyzing social media texts for depression prediction is challenging, and findings may not apply to a broader population. Nevertheless, our findings offer valuable insights for future research.
Trial Registration: PROSPERO CRD42023427707; https://www.crd.york.ac.uk/PROSPERO/view/CRD42023427707
doi:10.2196/59002
Keywords
Introduction
Background
Depression is a highly prevalent mental illness affecting people of various ages worldwide. According to the World Health Organization, more than 350 million people live with depression [
]. Individuals with depressive symptoms encounter challenges in diverse areas of their lives, such as work, relationships, and social interactions [ ]. These challenges manifest as sleep problems, diminished energy, loss of interest in daily activities, feelings of worthlessness, trouble focusing, and recurrent suicidal thoughts [ ]. In clinical settings, depression is typically identified either through clinical diagnoses or using standardized measurement tools that rely on subjective patient responses. However, both of these methods have limitations. Factors such as the surrounding context, the patient’s mental condition at that time, the relationship between the clinician and patient, the patient’s current emotional state, the patient’s clinical experiences, and memory bias can all affect individuals’ responses [ ]. Additionally, people may not be aware of or be ashamed of their depression, which can reduce their willingness to seek professional help. Approximately 70% of individuals avoid seeking professional medical advice during the initial phase of depression [ ]. Furthermore, depression diagnosis using conventional approaches based on in-person discussions is expensive both financially and in terms of time; thus, these approaches may not be viable for specific individuals [ ]. Therefore, an efficient strategy that can help predict and diagnose depression early in large groups of people would be beneficial.Social media is regarded as a valuable tool for investigating psychological well-being because it provides access to behaviors, interests, thoughts, and emotions of individuals, all of which may offer insights into their mental health [
]. Depression has received considerable attention in studies on the relationship between mental health disorders and social media [ ]. Facebook has more than 2 billion registered users globally, with approximately 1.25 billion active daily users [ ]. Twitter (subsequently rebranded X), another popular social network, has approximately 328 million registered users, with approximately 100 million using the platform daily [ ]. Various studies used social media textual data to identify and predict depression and other mental health disorders, as textual data provide more meaningful information than visual data [ , - ]. Text-based prediction involves using text data to anticipate future events, trends, and behavior patterns. In the realm of depression, text-based analysis on social media involves scrutinizing user-generated content from platforms such as Twitter and Facebook to identify signs and symptoms of depression among users. This approach leverages various natural language process techniques and machine learning models. In text-based prediction, the linguistic characteristics of social media text are examined; in addition to the user’s actions on the platform, the language features of words, part-of-speech tags, and n-grams can provide insights into the content and sentiment of web-based conversations [ ]. Using social media text to accurately predict depression can facilitate the identification of individuals who require a more comprehensive evaluation; they can then receive relevant resources, support, and treatment [ ]. Hence, more research is necessary on using social media textual data for predicting depression.Based on changes in features such as social media activity, language, and temporal characteristics, accurate statistical models for predicting depression can be developed. Social media textual analysis using traditional statistical methods has limited accuracy because of the unstructured nature of the input data. By contrast, machine learning can effectively examine nonlinear data, rendering it a superior option for analyzing social media data. The use of machine learning to predict depression on social media has increased substantially, as has the range of algorithms used for this purpose [
]. Machine learning can involve either shallow or deep learning, and the success of these methods varies according to the specific task [ ]. Machine learning has revolutionized clinical diagnostics, substantially improving prediction and diagnostic capabilities in clinical settings. Therefore, the integration of machine learning techniques in analyzing social media text data holds significant promise for advancing the prediction of depression.In machine learning-based prediction using textual data, the most relevant features must be extracted from the text to obtain an accurate model. This methodology is regarded as superior to other feature extraction approaches, as it exhibits a higher degree of independence from the system. Consequently, extracting features from text offers considerable flexibility and accuracy [
]. Demographic, language, social media activity, and temporal characteristics are the most common features adopted in prediction studies. Demographic features refer to the age, sex, and geographical location of individuals. A meta-analysis that considered language, social media activity, user demographics, and visual data revealed that demographic features were the most significant predictors of individual characteristics [ ]; however, that study assessed the link between social media and personality. Another study also indicated the potential of demographic features for predicting depression using machine learning algorithms; however, social media data were not used [ ]. Most social media platforms include user profiles with demographic information that can be used for depression prediction [ ]. Thus, assessing the role of demographics in depression prediction is vital.Language features represent how individuals use words across various categories, such as first-person singular pronouns, words relating to emotions (positive or negative) or sentiment, and depression-related words [
- ]. Studies revealed that people with and without depression have distinct linguistic styles on social media [ ], and people who frequently use words related to anxiety are more likely to experience depressive symptoms [ ]. A review study also highlighted a relationship between language features and depression; however, the effect was small [ ]. Therefore, understanding language features can enhance the accuracy of depression prediction, given their usefulness in identifying depression patterns.Feature extraction related to social media activity is also valuable for depression prediction. Social media activity features include metrics such as post frequency (daily or weekly), type of content shared, number of words, ratio of posts with URLs, number of friends, number of users following or followed, interactions with friends (likes or comments), and retweet ratio [
, - ]. Other key activity-related features are the relative volume of posts and reciprocity, which refers to liking and commenting on posts and retweeting and tweeting [ ]. The number of likes, comments, and retweets may serve as social attention and stress levels [ ]. Studies reported that users who are depressed tend to have a lower volume of posts and reciprocity than users who are not depressed [ , ]. Thus, evaluating the role of social media activity features in depression prediction is crucial.Furthermore, using temporal features in machine learning can lead to more accurate prediction models for identifying vulnerable individuals on social media. Temporal features refer to various time-related factors, including the user’s status during specific times and the frequency of posts each day. The timing pattern can indicate symptoms of insomnia in depressed individuals. One study reported that temporal features did not contribute to the prediction accuracy of models [
]. In contrast, another study reported that such features improved the accuracy of a model predicting depression based on Twitter data [ ]. Limited information is available on the role of temporal features in depression prediction; hence, additional research is required. In summary, studies adopted various features related to social media texts for predicting depression. However, further research is necessary to clarify the usefulness of demographic, social media activity, language, and temporal features in depression prediction.Research Problem and Aim
Several studies have reviewed the role of social media textual data in depression prediction [
, , , - ], but the overall evidence remains limited. Previous research often focused on specific features or had a limited scope regarding database searches, and studies included [ , ]. Therefore, in this study, we determine the effectiveness of user-generated social media texts in predicting depression using machine learning. Additionally, we evaluated the influence of demographic, language, social media activity, and temporal features in predicting depression on social media text using machine learning. Our research questions were as follows: How effective are social media texts in predicting depression? Moreover, what is the impact of demographic, language, social media activity, and temporal features in predicting depression using social media texts?Methods
Study Design
We followed the Meta-Analysis of Observational Studies in Epidemiology (MOOSE) guidelines [
] ( ) and registered our review on PROSPERO (CRD42023427707). There were no deviations from the registered protocol.Literature Search
Search terms were selected following the population, prediction factors, and outcome format for conducting systematic reviews for prognostic or prediction studies [
]. Our target population was social media users, prediction factors were prediction terms (ie, machine learning, algorithms, text mining, and language style), and the outcome was depression. We systematically searched for relevant studies on CINHAL (through EBSCOhost), PubMed, Scopus, Ovid MEDLINE, Embase, PubPsych, Cochrane Library, Web of Science, ProQuest, IEEE Explore, and ACM digital library. displays the search strings used in the study. Studies published from January 2008 to August 2023 were retrieved. This date range was selected because research on social media only gained prominence from 2008 onwards [ ]. Additional relevant studies were identified by manually searching the reference lists of the included studies and other review studies. Authors of the relevant studies that could not be accessible were contacted through email. Endnote version 20 was used to screen the searched studies [ ].Inclusion and Exclusion Criteria
We included studies that (1) used social media texts to investigate depression, (2) used machine learning, and (3) reported effect sizes (area under the curve, Pearson r, and specificity and sensitivity or data used to impute these values) for depression prediction. We excluded protocol papers and studies that were not written in English.
Screening and Selection of Relevant Studies
Two independent researchers (DP and FM) screened and selected the relevant studies by the inclusion and exclusion criteria. A third researcher (YAVP) was consulted to resolve disagreements. Duplicates were removed, and the remaining studies were evaluated through title and abstract screening. Thereafter, the full texts of potentially eligible studies were screened to identify relevant studies for further analysis.
Data Extraction
Two independent researchers (DP and FM) extracted the following information from the included studies: (1) study characteristics (publication year, sample size, and data points), (2) population characteristics (demographics and social media platform), (3) outcome measures (eg, Beck Depression Inventory [BDI], Center for Epidemiologic Studies Depression Scale [CES-D], Patient Health Questionnaire-9 [PHQ-9], Depression, Anxiety, and Stress Scales-21 items) or diagnostic framework (eg, Diagnostic and Statistical Manual of Mental Disorders), and (4) prediction factors (prediction features, algorithm models, and predictor values [area under the curve, Pearson r, and specificity and sensitivity or data used to impute these values]). For studies that reported area under the receiver operating characteristic curve statistics, we first converted the values to Cohen d, which were then converted into r [
, ]. Furthermore, for studies that provided sufficient information to compute sensitivity and specificity, we used this information to calculate odds ratios [ ], which were then converted into r values by using Comprehensive Meta-Analysis (version 3; Biostat, Inc). Effect sizes, such as Pearson r, allow for comparison across different studies and contexts, contributing to a more general understanding of the relationship between social media text, features, and depression [ ]. This is particularly important in meta-analyses, where combining and comparing findings from studies with varying methodologies and populations is crucial [ ]. Effect sizes provide a standardized way to do this, making it easier to draw broader conclusions. Furthermore, in many studies, particularly those included in our review, predictive performance metrics are not consistently measured, making it challenging to compare these metrics. By focusing on effect sizes, we ensure that we can consistently assess and compare the core findings related to depression prediction.The overall effect size was included for studies testing models that incorporated a set of features. In addition, for studies that compared the depression prediction performance of several models based on the same features but employed different algorithms, we extracted the effect size of the model with the optimal performance. Finally, the highest effect size was extracted for studies that did not reveal the effect size of each analyzed feature [
]. Any disagreement between the 2 researchers was resolved by consultation with a third reviewer (VLA).Data Analysis
Comprehensive Meta-Analysis version 3 software was used to run the meta-analysis. We adopted a random effects model to assess the uncertainty caused by variations between studies and report the pooled effect size from each study [
]. We used Pearson r to determine the relationship between social media texts and depression. Pearson r is classified into small (r=0.1), medium (r=0.3), and large (r=0.5) effect sizes [ ]. Article heterogeneity was determined using (1) the chi-square Q statistic, where P<.05 indicates significant heterogeneity, and (2) the I2 statistic, which represents the extent of variation. An I2 value of 0% indicates the absence of heterogeneity, and the I2 values of 1% to 25%, 25% to 75%, and more than 75% indicate low, moderate, and high heterogeneity, respectively [ ]. The features adopted in the reviewed studies were grouped into demographic, language, social media activity, and temporal features to assess their overall effect on depression prediction. In addition, we performed a moderator analysis with a random effects model to identify the source of heterogeneity [ ]. We included the following groups: social media platform (public and private) [ , ], machine learning (shallow and deep), model validation (10-fold and other), participant sample size (<1000 and ≥1000), data points (<100,000 and ≥100,000), publication (journal and proceedings), features (single and multiple), and use of outcome measures (yes and no). Finally, we conducted a sensitivity analysis to determine the stability of the results [ ]. We used a leave-one-out method to examine the effect of each study on the overall effect.Publication Bias
We generated a funnel plot to examine the presence of publication bias in the included studies [
]. Statistical evaluation was performed using the Begg-Mazumdar rank correlation and Egger tests [ ]. A P value of <.05 denotes the presence of significant publication bias. For studies with publication bias, we calculated the adjusted estimated effect sizes by using Duval and Tweedie trim and fill test, with consideration of the potential effect of missing studies [ ].Results
Paper Selection Protocol
The flowchart of study screening and selection is presented in
. The electronic database search yielded 6278 studies, from which we excluded 1285 duplicates. We screened the abstracts and titles of 4993 studies and excluded 4841 studies that did not meet the eligibility criteria. We conducted full-text screening on 152 eligible studies and excluded studies that did not report social media use (n=24), were protocols (n=6), and had incomplete information to compute effect sizes (n=90). The remaining 32 studies were included in our meta-analysis. Search by citation yielded seven studies, three of which were excluded due to the lack of appropriate information for computing the effect size. The remaining 4 studies were added to the 32 studies, yielding 36 for analysis. provides a list of the included studies.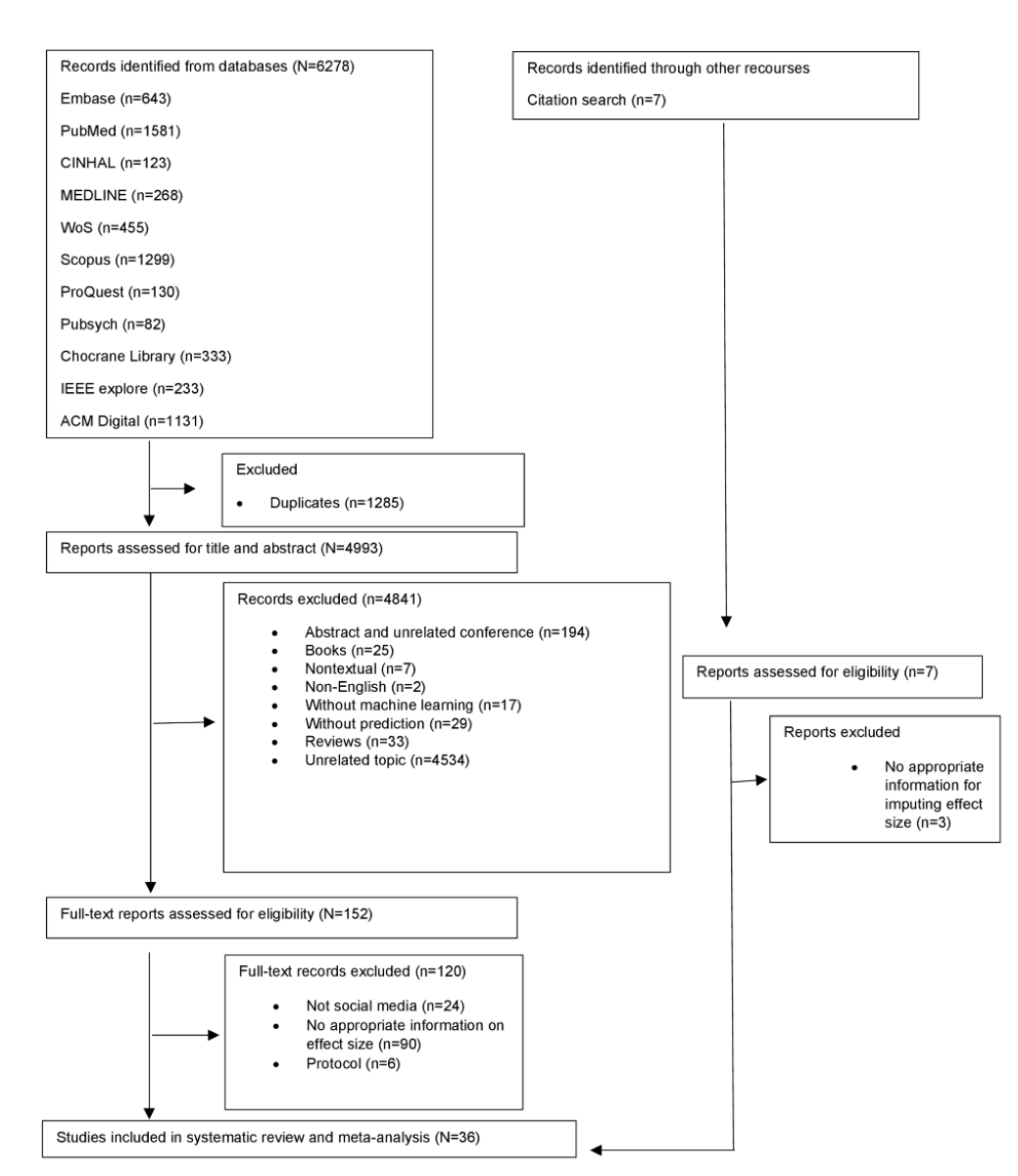
Characteristics of Included Studies
The revised PRISMA (Preferred Reporting Items for Systematic Reviews and Meta-Analyses) checklist is provided in
, and the characteristics of the included studies are outlined in . summarizes the key information of the included studies. All of the included studies were published between 2013 and 2023; 12 were published in journals and 24 in conference proceedings. Eighteen studies reported the sample size, ranging from 50 to 344,657 (total: 3,969,013). Seven of these studies reported the sex distribution of participants (total participants: 24,502) with 58% identified as females while the remaining 11 papers did not provide information on the sex distribution of the participants. Twitter (n=18, 50%) was the most common platform investigated, followed by Facebook (n=7, 19.4%), Reddit (n=5, 13.9%), Sina Weibo (n=2, 5.6%), Instagram (n=2, 5.6%), Vkontakte (n=2, 5.6%), and Live Journal (n=1, 2.8%). Regarding the machine learning approach, 25 studies adopted shallow learning algorithms (eg, support vector machine, BayesNet, random forest, linear regression, k-nearest neighbor, naïve Bayes, and decision tree), whereas 11 studies used deep learning algorithms (eg, deep neural network, long short-term memory, and convolutional neural network). Eight studies applied 10-fold cross-validation, whereas 9 studies used other types of validation, namely leave-one-out, hold-out, and binary classification validation, as well as 4-fold, 5-fold, and 20-fold cross-validation. Sixteen studies used outcome measures or diagnostic frameworks (Depression, Anxiety, and Stress Scales, CES-D, PHQ-9, PHQ-8, BDI, and Diagnostic and Statistical Manual of Mental Disorders), whereas 20 used participants’ diagnostic statements (eg, “I was diagnosed with depression”) or did not provide relevant information. Language features (n=17, 85%) were the most commonly examined features, followed by social media activity (n=8, 40%), temporal (n=4, 20%), and demographic (n=3, 15%) features.Characteristics | Studies, n (%) | Participants, n (%) | |||
Data points | 29 (80.6) | —a | |||
Participants sample size | 18 (50) | 396,901 (100) | |||
Sex | 7 (44.4) | 24,502 (6.2) | |||
Male | — | 10,286 (42) | |||
Female | — | 14,216 (58) | |||
Social media platform | 36 (100) | — | |||
18 (50) | — | ||||
7 (19.4) | — | ||||
5 (13.9) | — | ||||
Sina Weibo | 2 (5.6) | — | |||
2 (5.6) | — | ||||
Vkontakte | 2 (5.6) | — | |||
LiveJournal | 1 (2.8) | — | |||
Machine learning approach | 36 (100) | — | |||
Shallow | 25 (69.4) | — | |||
Deep | 11 (30.6) | — | |||
Model validation | 17 (47.2) | — | |||
10-fold | 8 (47.1) | — | |||
Other validationsb | 9 (52.9) | — | |||
Use of outcome measures | 36 (100) | — | |||
Yes | 16 (44.4) | — | |||
No | 20 (55.6) | — | |||
Publication type | 36 (100) | — | |||
Journal | 12 (33.3) | — | |||
Proceeding | 24 (66.7) | — | |||
Features | 20 (55.5) | — | |||
Demographics | 3 (15) | — | |||
Social media activity | 8 (40) | — | |||
Language | 17 (85) | — | |||
Temporal | 4 (20) | — |
aNot applicable.
bLeave-one-out, hold out, and binary classification validation; 4-fold, 5-fold, and 20-fold cross-validations.
The Effectiveness of Social Media Text in Predicting Depression
The 36 included studies [
- ] indicated a large significant overall effect size of r=0.630 (95% CI 0.571-0.693), indicating the effectiveness of the user-generated social media text in predicting depression. Significant heterogeneity was observed between the studies (Q=810 038.087; I2=99.996; P<.001; [ - ]).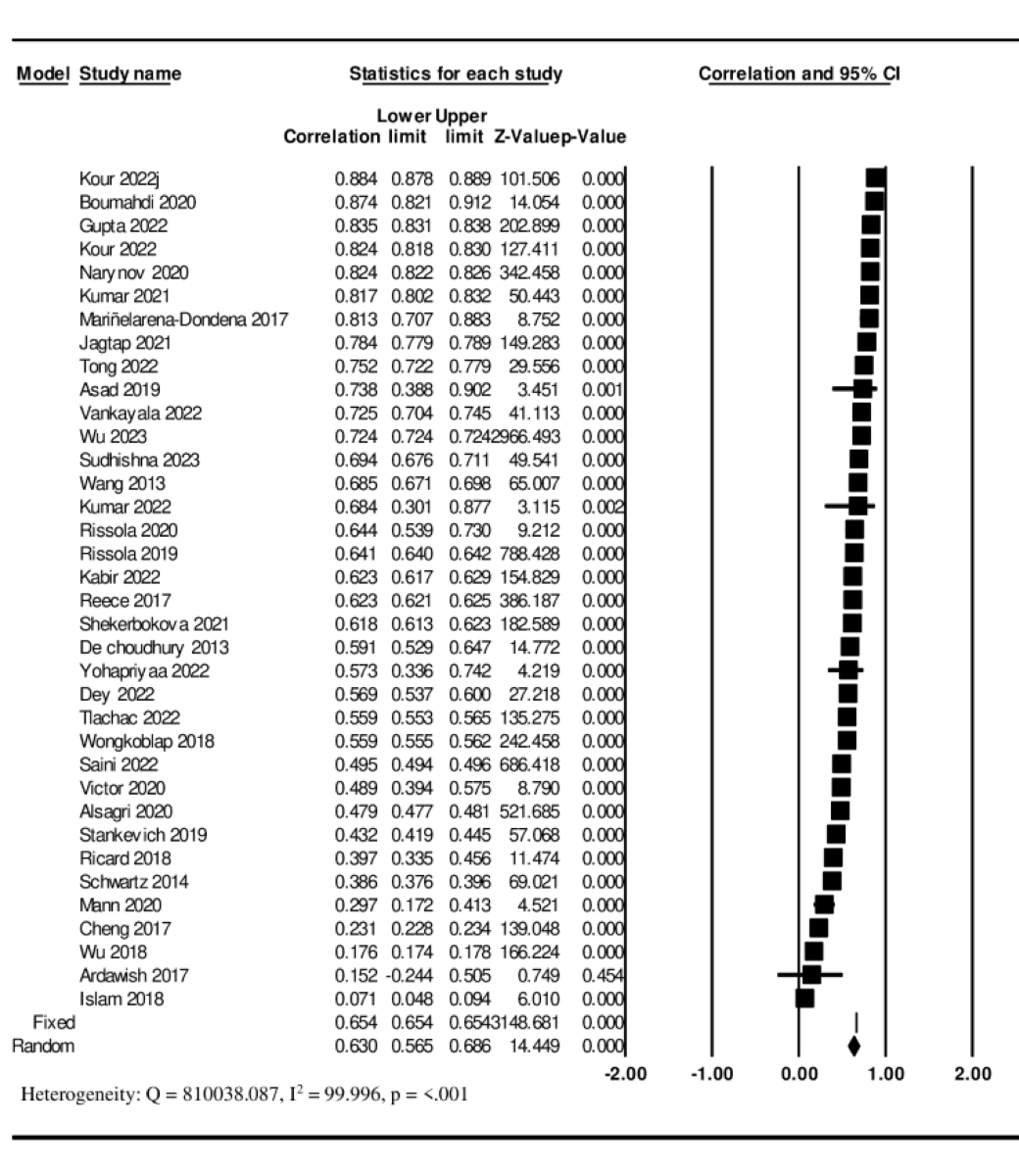
The Influence of Features in Predicting Depression on Social Media Texts
The findings revealed that all 4 included features; demographic, social media activity, language, and temporal had significant large effect sizes (r=0.642; 95% CI 0.489-757; r=0.552, 95% CI 0.418-0.663; r=0.545, 95% CI 0.434-0.639; and r=0.531, 95% CI 0.320-0.693; respectively). A high significant heterogeneity was observed between the studies (I2=98%-99.9%).
provides detailed results, and forest plots are presented in [ , , , , - , , , , , , , , , - ].Feature | Features, n (%) | r (95% CI) | Q | I2 | P value |
Demographic | 3 (15) | 0.642 (0.489-0.757) | 109.732 | 98.177 | <.001 |
Social media activity | 8 (40) | 0.552 (0.418-0.663) | 96346.650 | 99.993 | <.001 |
Linguistic | 17 (85) | 0.545 (0.434-0.639) | 782874.071 | 99.998 | <.001 |
Temporal | 4 (20) | 0.531 (0.320-0.693) | 198014.084 | 99.998 | <.001 |
Moderator Analysis
Of all the categories contributing to heterogeneity, only social media platform type, machine learning approach, and use of outcome measures significantly accounted for the observed heterogeneity, with P values of <.001, .048, and <.001, respectively (
).Category | n (%) | Point estimate (95% CI) | P value | ||||
Social media platform | 33 (100) | —a | <.001 | ||||
Public | 28 (84.8) | 0.674 (0.618-0.723) | <.001 | ||||
Private | 5 (15.2 | 0.368 (0.166-0.540) | .001 | ||||
Machine learning approach | 36 (100) | — | .048 | ||||
Shallow | 25 (69.4) | 0.584 (0.491-0.663) | <.001 | ||||
Deep | 11(30.6) | 0.719 (0.610-0.801) | <.001 | ||||
Model validation | 17 (100) | — | .434 | ||||
10-fold cross-validation | 8 (47.1) | 0.522 (0.349-0.660) | <.001 | ||||
Other validationsb | 9 (52.9) | 0.602 (0.458-0.715) | <.001 | ||||
Participant sample size | 18 (100) | — | .677 | ||||
<1000 | 11 (61.1) | 0.571 (0.423-0.690) | <.001 | ||||
≥1000 | 7 (38.9) | 0.525 (0.330-0.677) | <.001 | ||||
Data points | 29 (100) | — | .627 | ||||
<100,000 | 19 (65.5) | 0.650 (0.563-0.723) | <.001 | ||||
≥100,000 | 10 (34.5) | 0.616 (0.486-0.719) | <.001 | ||||
Publication type | 36 (100) | — | .824 | ||||
Journal | 12 (33.3) | 0.637 (0.554-0.707) | <.001 | ||||
Proceedings | 24 (66.6) | 0.626 (0.565-0.680) | <.001 | ||||
Features | 19 (100) | — | .853 | ||||
Single | 8 (42.1) | 0.552 (0.362-0.698) | <.001 | ||||
Multiple | 11 (57.9) | 0.536 (0.393-0.652) | <.001 | ||||
Use of outcome measure | 36 (100) | — | <.001 | ||||
Yes | 16 (44.4) | 0.472 (0.381-567) | <.001 | ||||
No | 20 (55.6) | 0.722 (0.667-769) | <.001 |
aNot applicable.
bLeave-one-out, hold out, and binary classification validation; 4-fold, 5-fold, and 20-fold cross-validation
Sensitivity Analysis
The results revealed that the overall effect size did not change (r=0.630, 95% CI 0.571-0.693) after performing a leave-one-study-out sensitivity analysis method, and the heterogeneity between the studies was significant (Q=810038.087; I2=99.996; P<.001;
[ - ]).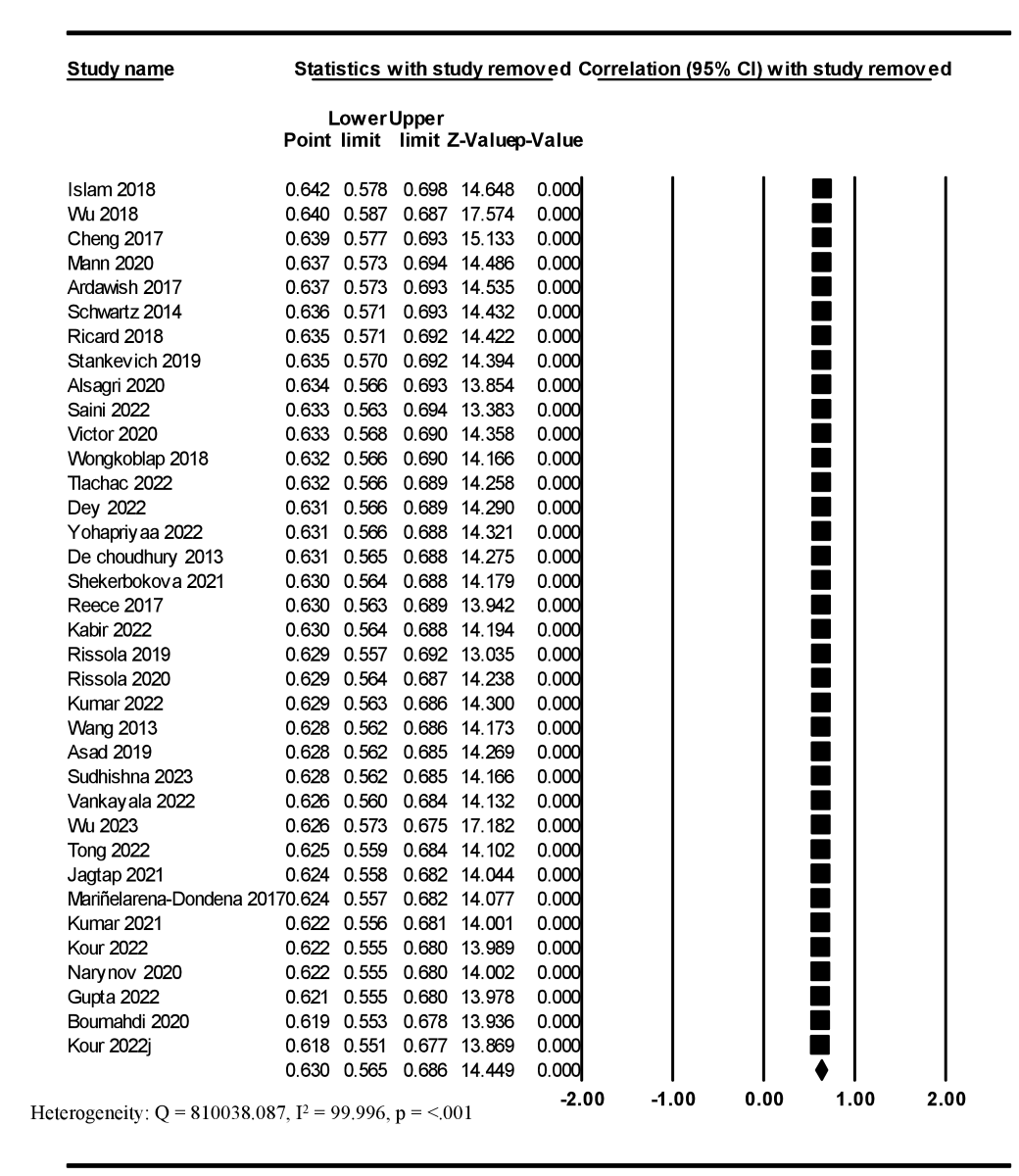
Publication Bias
presents the funnel plot used to inspect publication bias visually. The nonsignificant results of the Begg–Mazumdar rank correlation test (Kendall τb=0.22063; P=.058) and Egger test (2-tailed t34=1.28696; P=.21) indicated the absence of publication bias. The Duval and Tweedie trim and fill test revealed no studies were trimmed [ ].
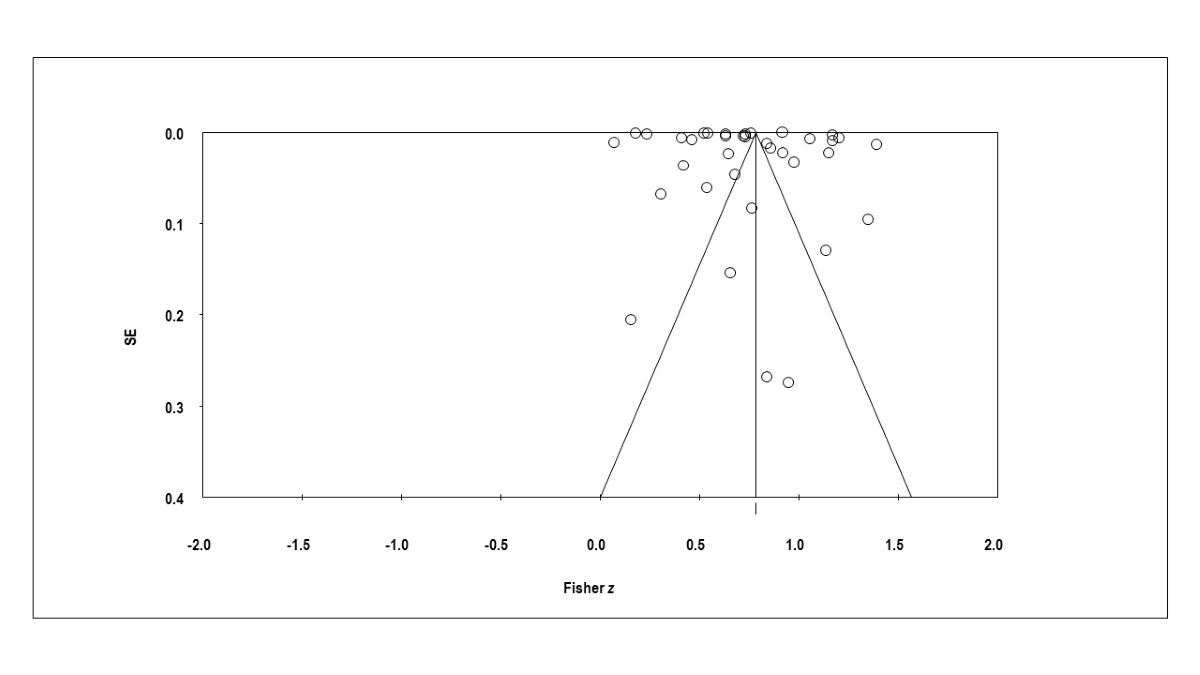
Discussion
Principal Findings
This systematic review and meta-analysis examined the effect size of social media texts and the influence of demographics, social media activity, language, and temporal features on depression prediction using machine learning. We observed a significantly large overall effect size for social media texts on depression, indicating the capability of social media texts to predict depression. The demographics, social media activity, language, and temporal features also exhibited significantly large effect sizes, indicating their influence in predicting depression on social media texts. Demographic features had the largest effect size of all the features. Thus, demographic features merit special attention to improve the accuracy of depression prediction models based on social media data. Our results also indicated that the social media platform type (public or private), machine learning approach (shallow or deep), and use of outcome measures (yes or no) were significant moderator variables. Therefore, these factors should be considered in the prediction of depression using social media data. Overall, our findings provide solid evidence that social media texts can predict depression.
Comparison With Prior Work
The extracted effect size of the social media texts on depression prediction in our study was larger than that reported in a related study (r=0.37) between digital traces and psychological well-being [
]. Our results strengthen the growing evidence that social media texts can serve as a valuable means of monitoring and predicting depression. Overall, social media data have considerable potential to advance health research and enhance the capacity to address mental health concerns. Individuals with depression, particularly teenagers and young adults, commonly use social media to express their emotions [ ]. Research has revealed that in large prediction models, identifying individuals with depression is more challenging when using social media data than when using electronic health records [ ]. However, social media post analysis can yield valuable insights into users’ daily events, activities, and interests [ ]. An examination of the language, sentiment, and emotions in these posts can provide information on user behavior, mood, socialization, and opinions [ ]. For instance, emotions such as helplessness, worthlessness, and self-hatred in a user’s posts may indicate depression [ ]. Therefore, social media is a valuable tool for obtaining a comprehensive overview of a user’s mental health and identifying those with depression.Our results revealed that demographic, social media activity, temporal, and language features had an influence on depression prediction, with demographic features having the largest effect size. The use of social media textual features in machine learning has proven useful for predicting depression, without any preconceived notions [
]. A related study indicated that the incorporation of demographic data can considerably enhance the accuracy of models linking profile attributes, images, and depression [ ]. Similarly, another study reported that demographics are a crucial factor in depression prediction on social media [ ]. A study also revealed a significant association between negative emotions and anger and depression among individuals aged 18-25 years on Facebook; however, that study did not use machine learning algorithms [ ]. Notably, we analyzed only 3 studies that adopted demographic features, potentially limiting the generalizability of our results. Nevertheless, the results provide strong evidence that demographic data can substantially enhance the prediction value of social media text for depression.A previous study revealed that the social media activity features on Twitter could predict depression with an accuracy of 69% [
]. Another study, using traditional statistical methods, reported both positive and negative relationships between individual social media activity features and depression on Facebook; individuals with depression exhibited a reduced number of likes and comments, and they posted on their own walls with greater frequency than did their counterparts without depression [ ]. Social media activity features can be used to gain valuable insights into individuals’ social media engagement. A reduced level of engagement, particularly a decline in the frequency of their posts, may signify a loss of social connectedness, which has been associated with depressive symptoms [ ]. Social media activity features can thus serve as a valuable supplement to self-reported information and clinical evaluations, justifying their inclusion in depression prediction models.In our study, language features also had a large effect on depression prediction on social media texts. Language features tend to be the most crucial for predicting depression on social media using machine learning. Consistent with our results, a study on depression prediction using social media data and medical records revealed that the strongest predictor of depression was the use of words related to rumination, loneliness, and hostility [
]. Additionally, researchers have suggested that using first-person singular pronouns is associated with depression and suicidal behavior [ , ]. A significant link has also been reported between negative emotions and depression on Facebook; however, the effect was small, and machine learning was not adopted [ ]. These findings emphasize the pivotal role of language style in predicting depression on social media.Our results indicated that temporal features had a large effect on the prediction of depression on social media. By contrast, a related study reported that temporal features did not provide valuable additional user-specific information that could enhance the accuracy of the depression prediction model [
]. The temporal patterns of web-based posts can offer useful insights into the social context and communication styles of individuals [ ]; for example, individuals with depression may experience irregular sleep patterns and thus post on social media late at night or early in the morning [ ]. Furthermore, consistent with our results, a study that considered temporal measures on Twitter reported that monitoring changes in an individual’s emotional state over time can aid in identifying those who may be experiencing depression [ ]. Temporal features may provide the necessary context and help differentiate between a temporary emotional state and a long-term mental health problem. For instance, a single sad post is unlikely to indicate depression, whereas regular sad posts over time suggest a deeper problem that merits attention. Therefore, the inclusion of temporal features can increase the accuracy of depression prediction models based on social media data.Our findings indicated that the social media platform type, machine learning approach, and use of outcome measures were significant moderators of the relationship between textual features and depression prediction. Most of the studies included in our review focused on public social media platforms, with Twitter being the most common target. Our findings are inconsistent with those of a previous study that reported that the social media platform type had no mediating effect [
]. Although public social media platforms, such as Twitter, cater to diverse user bases and offer varied experiences [ ], research has indicated that the use of private social media platforms, such as Facebook, is linked with depressive symptoms, principally because of the loss of real-world social interaction [ ]. Further research is thus necessary on the impact of social media platform type on depression prediction.In our study, the adopted machine learning approach had a significant impact on the relationship between social media text and depression prediction. One study also reported that the accuracy of depression prediction based on textual data depends on the type of model used [
]. Most of the studies included in our analysis adopted shallow machine learning, which involves the use of features such as language style and emotional language as training data for developing a depression prediction model [ ]. In general, shallow machine learning models consist of neural networks with only 1 hidden layer. By contrast, deep learning models use neural networks with multiple hidden layers [ ]. Shallow learning models typically outperform deep learning in terms of speed and ease of use, but deep learning models can be used to investigate complex phenomena [ , ]. The efficacy of both deep and shallow learning methods depends on the specific application [ ]. When limited data are available, training complex neural networks is impossible because these networks require the determination of a large number of parameters from the data; shallow networks are the only option in such cases [ ]. Additionally, deep learning models, such as recurrent neural networks, encounter difficulty in identifying the proper context in long sentences [ ]. The use of machine learning techniques has contributed considerably to enhancing prediction and diagnostic capabilities in clinical settings [ ]. On the basis of unstructured data, this approach can generate insights that can help identify high-risk conditions, facilitating early treatment and improving patient outcomes.In this review, 20 of the 36 studies did not specify the tools or instruments that were used for depression measurement, or they used subjective patient reports. This could be attributed to many of the studies being performed on public platforms, which is a more cost-effective approach for collecting data from a large sample. A substantial amount of publicly available data are self-reported statements regarding depression diagnoses [
]. Although this approach can yield valuable insights, the findings may have limited accuracy. To ensure reliable and accurate results, the use of survey-based measures, such as CES-D, PHQ-9, or BDI, is paramount, although higher costs would be incurred [ ]. The studies included in this review adopted various outcome measures; to elucidate the effectiveness and contribution to the overall prediction accuracy of these tools, a comprehensive examination is imperative.Strengths and Limitations
Our study has several strengths. First, to the best of our knowledge, this is the first systematic review and meta-analysis of the effectiveness of user-generated social media texts on depression prediction; our study thus expands depression research. Second, we observed a significantly large overall effect of social media texts on depression prediction, providing strong evidence for the accuracy of social media data in predicting depression. Third, the social media platform, demographic, social media activity, language, and temporal features in this study exhibited a large and more significant influence on depression prediction than similar features in previous studies, providing insights into improving screening and prevention efforts. Fourth, unlike previous reviews, we searched 11 databases, ensuring our search was robust and comprehensive. Fifth, we included 36 studies supporting the reliability of our findings. Previous studies included only 2 [
] and 4 [ ] studies, respectively. Sixth, our moderator analysis revealed that the type of machine learning approach affects the accuracy of depression prediction using social media data. Previous reviews did not consider this factor. Seventh, in contrast to previous studies, our sensitivity analysis confirmed the robustness of our findings. This analysis reaffirms the validity of our research and strengthens the credibility of our conclusions.Despite the strengths and novelty of our study, some limitations should be noted. First, although social media texts are preferred for depression prediction, such data can be more challenging to analyze than survey data because of the considerable variability in the frequency and length of social media posts as well as the likelihood of these variables changing over time. Second, findings based on social media texts cannot be generalized to a wider population because age, income, education, and ethnicity tend not to be proportionately represented in the data. Third, half of the included studies contained insufficient information on the overall effects of the four target features in predicting depression. Fourth, we could not analyze the effects of specific demographics, language, temporal, and social media activity features. Fifth, most of the studies failed to provide information on model validation, a key step for enhancing the accuracy and effectiveness of machine learning. Sixth, our study was limited to English-only studies because reviewing and interpreting studies in unfamiliar languages may lead to misinterpretation and errors in data extraction and analysis. Seventh, many of the included studies were from proceedings, and because most conference papers are not peer-reviewed, the quality of the studies may be in question. Eighth, we could not evaluate the quality of the included studies due to a lack of appropriate guidelines. Ninth, we acknowledge the omission of other keywords like “Reddit” and “classification,” which limits the comprehensiveness of our search strategy. However, considering the novelty of this research area, our findings provide valuable insights that can form the basis of future research. Future studies may explore social media visual data along with specific features within each category (ie, demographic, language, social media activity, and temporal features) and machine learning algorithms for depression prediction on social media. Moreover, it is essential for future research to incorporate a broader range of keywords, including “Reddit” and “classification,” to enhance the search strategy and capture relevant studies effectively.
Conclusions
Our findings revealed that social media text has a significantly large overall effect on depression prediction using machine learning. Specifically, demographic features had a larger effect size than social media activity, language, and temporal features. Furthermore, the social media platform type, use of outcome measures, and machine learning approach were significant moderators. Our results suggest that social media texts are effective in predicting depression. Social media textual content, particularly demographic features, is thus useful for predicting depression on social media texts. Finally, the social media platform type, machine learning approach used, and use of outcome measures can affect the accuracy of predictions. Our findings may help mental health and psychiatric practitioners identify individuals who require further evaluation; these individuals can receive the necessary resources, support, and treatment.
Acknowledgments
The authors acknowledge Wallace Academic Editing for editing this manuscript.
Data Availability
Data availability is not applicable to this article as no new data were created or analyzed in this study.
Authors' Contributions
DP originated the study idea and wrote the initial draft of the manuscript, developed the search strategy, identified and screened the studies, extracted data from individual studies, and conducted a systematic review and meta-analysis using Comprehensive Meta-Analysis software, FM conducted screening of the studies, extracting data as the second researcher, and reviewing the manuscript draft, VLA contributed to data extraction as the third researcher in cases where DP and FM had disagreements, evaluated the studies and helped create forest plots for the meta-analysis, YVAP was involved in manually searching for studies, screening them, reviewing the final draft, and editing the manuscript, LPD Assisted in formulating the search strategy, contributed to subgroup and meta-regression analyses, and reviewed and edited the manuscript, MHC contributed to the developing of the study idea, wrote the final draft of the manuscript, and provided significant review of tables and editing of the manuscript. All authors reviewed the final manuscript.
Conflicts of Interest
None declared.
MOOSE (Meta-Analysis of Observational Studies in Epidemiology) checklist for meta-analyses of observational studies.
PDF File (Adobe PDF File), 122 KBSearch strategy.
DOCX File , 20 KBList of included studies.
DOCX File , 18 KBRevised PRISMA (Preferred Reporting Items for Systematic Reviews and Meta-Analyses) checklist.
PDF File (Adobe PDF File), 117 KBStudy characteristics.
DOCX File , 24 KBForest plots of the influence of demographic, social media activity, language, and temporal features in predicting depression.
DOCX File , 1149 KBReferences
- Marcus M, Yasamy MT, van Ommeren MV, Chisholm D, Saxena S. Depression: A global public health concern. World Health Organization. 2012. URL: https://www.researchgate.net/publication/285075782_Depression_A_global_public_health_concern [accessed 2024-07-16]
- Almouzini S, Khemakhem M, Alageel A. Detecting Arabic depressed users from Twitter data. Procedia Comput Sci. 2019;163:257-265. [CrossRef]
- American Psychiatric Association. Diagnostic and Statistical Manual of Mental Disorders (DSM-5-TR). Washington, DC. American Psychiatric Association; 2013.
- Liu D, Feng XL, Ahmed F, Shahid M, Guo J. Detecting and measuring depression on social media using a machine learning approach: systematic review. JMIR Ment Health. 2022;9(3):e27244. [FREE Full text] [CrossRef] [Medline]
- Shen G, Jia J, Nie L, Feng F, Zhang C, Hu T. Depression detection via harvesting social media: a multimodal dictionary learning solution. 2017. Presented at: IJCAI'17: Proceedings of the 26th International Joint Conference on Artificial Intelligence; Melbourne, Australia; August 19-25, 2017. URL: https://doi.org/10.24963/ijcai.2017/536 [CrossRef]
- Salas-Zárate R, Alor-Hernández G, Salas-Zárate MDP, Paredes-Valverde M, Bustos-López M, Sánchez-Cervantes JL. Detecting depression signs on social media: a systematic literature review. Healthcare. 2022;10(2):219. [FREE Full text] [CrossRef] [Medline]
- Chancellor S, De Choudhury M. Methods in predictive techniques for mental health status on social media: a critical review. NPJ Digit Med. 2020;3:43. [FREE Full text] [CrossRef] [Medline]
- Azucar D, Marengo D, Settanni M. Predicting the Big 5 personality traits from digital footprints on social media: a meta-analysis. Personal Individ Differ. 2018;124:150-159. [FREE Full text] [CrossRef]
- William D, Suhartono D. Text-based depression detection on social media posts: a systematic literature review. Procedia Comput Sci. 2021;179:582-589. [FREE Full text] [CrossRef]
- Chiong R, Budhi GS, Dhakal S, Chiong F. A textual-based featuring approach for depression detection using machine learning classifiers and social media texts. Comput Biol Med. 2021;135:104499. [CrossRef] [Medline]
- Verma JP, Agrawal S. Big data analytics: Challenges and applications for text, audio, video, and social media sata. Int J Soft Comput Artif Intell Appl. 2016;5(1):41-51. [FREE Full text] [CrossRef]
- Zhang Z, Chen S, Wu M, Zhu KQ. Symptom identification for interpretable detection of multiple mental disorders. ArXiv. Preprint posted online on May 23, 2022. 2022. [FREE Full text]
- Guntuku SC, Yaden DB, Kern ML, Ungar LH, Eichstaedt JC. Detecting depression and mental illness on social media: an integrative review. Curr Opin Behav Sci. 2017;18:43-49. [FREE Full text] [CrossRef]
- Robles Herrera S, Ceberio M, Kreinovich V. When is deep learning better and when is shallow learning better: qualitative analysis. Int J Parallel Emergent Distrib Syst. 2022;37(5):589-595. [FREE Full text] [CrossRef]
- Budhi GS, Chiong R, Wang Z. Resampling imbalanced data to detect fake reviews using machine learning classifiers and textual-based features. Multimed Tools Appl. 2021;80(9):13079-13097. [FREE Full text] [CrossRef]
- Settanni M, Azucar D, Marengo D. Predicting individual characteristics from digital traces on social media: a meta-analysis. Cyberpsychol Behav Soc Netw. 2018;21(4):217-228. [FREE Full text] [CrossRef] [Medline]
- Sun J, Liao R, Shalaginov MY, Zeng TH. A machine-learning approach for predicting depression through demographic and socioeconomic features. 2022. Presented at: IEEE International Conference on Bioinformatics and Biomedicine (BIBM); Las Vegas, NV; December 6-8, 2022. URL: https://doi.org/10.1109/BIBM55620.2022.9994921 [CrossRef]
- Hussain J, Satti FA, Afzal M, Khan WA, Bilal HSM, Ansaar MZ, et al. Exploring the dominant features of social media for depression detection. J Inf Sci. Aug 12, 2019;46(6):739-759. [CrossRef]
- Tackman AM, Sbarra DA, Carey AL, Donnellan MB, Horn AB, Holtzman NS, et al. Depression, negative emotionality, and self-referential language: a multi-lab, multi-measure, and multi-language-task research synthesis. J Pers Soc Psychol. 2019;116(5):817-834. [CrossRef] [Medline]
- Tølbøll KB. Linguistic features in depression: a meta-analysis. J Lang Works. 2019;4:39-59. [FREE Full text]
- Zulkarnain N, Basiron H, Abdullah N. Writing style and word usage in detecting depression in social media: a review. J Theor Appl Inf Technol. 2020;98:124-135. [FREE Full text]
- Ramirez-Esparza N, Chung C, Kacewic E, Pennebaker J. The psychology of word use in depression forums in English and in Spanish: testing two text analytic approaches. Proc Int AAAI Conf Web Soc Media. 2021;2(1):102-108. [FREE Full text] [CrossRef]
- Seabrook EM, Kern ML, Rickard NS. Social networking sites, depression, and anxiety: a systematic review. JMIR Ment Health. 2016;3(4):e50. [FREE Full text] [CrossRef] [Medline]
- Tsugawa S, Kikuchi Y, Kishino F, Nakajima Y, Itoh Y, Ohsaki H. Recognizing depression from Twitter activity. 2015. Presented at: CHI '15:Proceedings of the 33rd Annual ACM Conference on Human Factors in Computing Systems; April 18-23, 2015:3187-3196; Seoul, South Korea. URL: https://doi.org/10.1145/2702123.2702280 [CrossRef]
- Lin H, Jia J, Guo Q, Xue Y, Li Q, Huang J, et al. User-level psychological stress detection from social media using deep neural network. 2014. Presented at: MM '14: Proceedings of the 22nd ACM international conference on Multimedia; November 3-7, 2014:507-516; Orlando, FL. URL: https://doi.org/10.1145/2647868.2654945 [CrossRef]
- Park S, Kim, I, Lee SW, Yoo J, Jeong B, Cha M. Manifestation of depression and loneliness on social networks: a case study of young adults on Facebook. 2015. Presented at: CSCW '15: Computer Supported Cooperative Work and Social Computing; March 14-18, 2015:557-570; Vancouver, BC. URL: https://doi.org/10.1145/2675133.2675139 [CrossRef]
- Vr'nak D, Paulinovic M, Kuzina V. Early Depression Detection Using Temporal and Sentiment Features. Zagreb, Croatia. University of Zagreb; 2019. URL: https://www.fer.unizg.hr/_download/repository/TAR-2019-ProjectReports.pdf#page=72 [accessed 2023-10-22]
- Chen X, Sykora MD, Jackson TW, Elayan S. What about mood swings: identifying depression on Twitter with temporal measures of emotions. 2018. Presented at: WWW '18: Companion Proceedings of the The Web Conference 2018; April 23-27, 2018:1653-1660; Lyon, France. URL: https://doi.org/10.1145/3184558.3191624 [CrossRef]
- Biswas S, Hasija Y. Predicting depression through social media. In: Mittal M, Goyal LM, editors. Predictive Analytics of Psychological Disorders in Healthcare. Singapore. Springer; 2022:109-127.
- Zhong M, Zhang H, Yu C, Jiang J, Duan X. Application of machine learning in predicting the risk of postpartum depression: a systematic review. J Affect Disord. 2022;318:364-379. [CrossRef] [Medline]
- Gupta GK, Sharma DK. Depression detection on social media with the aid of machine learning platform: a comprehensive survey. 2021. Presented at: 8th International Conference on Computing for Sustainable Global Development (INDIACom); March 17-19, 2021; New Delhi, India. URL: https://ieeexplore.ieee.org/document/9441093
- Brooke BS, Schwartz TA, Pawlik TM. MOOSE reporting guidelines for meta-analyses of observational studies. JAMA Surg. 2021;156(8):787-788. [CrossRef] [Medline]
- EndNote. Clarivate. 2013. URL: https://support.clarivate.com/Endnote/s/article/Citing-the-EndNote-program-as-a-reference?language=en_US [accessed 2023-12-05]
- Rosenthal R, Cooper H, Hedges L. Hedges, parametric measures of effect size. In: Cooper HM, Hedges LV, editors. The Handbook of Research Synthesis. Manhattan, NY. Russell Sage Foundation; 1994:231-244.
- Ruscio J. A probability-based measure of effect size: robustness to base rates and other factors. Psychol Methods. 2008;13(1):19-30. [CrossRef] [Medline]
- Glas AS, Lijmer JG, Prins MH, Bonsel GJ, Bossuyt PMM. The diagnostic odds ratio: a single indicator of test performance. J Clin Epidemiol. 2003;56(11):1129-1135. [CrossRef] [Medline]
- Lakens D. Calculating and reporting effect sizes to facilitate cumulative science: a practical primer for t-tests and ANOVAs. Front Psychol. 2013;4:863. [FREE Full text] [CrossRef] [Medline]
- Borenstein M, Hedges LV, Higgins JP, Rothstein HR, Ben Van Den A. Introduction to Meta-Analysis. Hoboken, NJ. John Wiley & Sons; 2020:121-122.
- Borenstein M, Hedges LV, Higgins JPT, Rothstein HR. A basic introduction to fixed-effect and random-effects models for meta-analysis. Res Synth Methods. 2010;1(2):97-111. [CrossRef] [Medline]
- Cohen J. Statistical Power Analysis for the Behavioral Sciences. Mahwah, NJ. Lawrence Erlbaum Associates; 1988.
- Higgins JPT, Thompson SG. Quantifying heterogeneity in a meta-analysis. Stat Med. 2002;21(11):1539-1558. [CrossRef] [Medline]
- Bown MJ, Sutton AJ. Quality control in systematic reviews and meta-analyses. Eur J Vasc Endovasc Surg. 2010;40(5):669-677. [CrossRef] [Medline]
- White CL, Boatwright B. Social media ethics in the data economy: issues of social responsibility for using Facebook for public relations. Public Relat Rev. 2020;46(5):101980. [FREE Full text] [CrossRef]
- Sterne JAC, Becker BJ, Egger M. The funnel plot. In: Rothstein HR, Sutton AJ, Borenstein M, editors. Publication Bias in Meta‐Analysis: Prevention, Assessment and Adjustments. Hoboken, NJ. Wiley; 2005:73-98.
- Sterne JAC, Egger M. Regression Methods to Detect Publication and Other Bias in Meta-Analysis. Hoboken, NJ. Wiley; 2005:99-110.
- Duval S. The trim and fill method. In: Rothstein HR, Sutton AJ, Borenstein M, editors. Publication Bias in Meta-Analysis: Prevention, Assessment and Adjustments. Hoboken, NJ. Wiley; 2005:127-144.
- Aldarwish MM, Ahmad HF. Predicting depression levels using social media posts. 2017. Presented at: 2017 IEEE 13th International Symposium on Autonomous Decentralized System (ISADS); March 22-24, 2017; Bangkok, Thailand. [CrossRef]
- Alsagri HS, Ykhlef M. Machine learning–based approach for depression detection in Twitter using content and activity features. IEICE Trans Inf Syst. 2020:1825-1832. [CrossRef]
- Asad NA, Mahmud Pranto MA, Afreen S, Islam MM. Depression detection by analyzing social media posts of user. 2019. Presented at: 2019 IEEE International Conference on Signal Processing, Information, Communication & Systems (SPICSCON); November 28-30, 2019; Dhaka, Bangladesh. [CrossRef]
- Cheng Q, Li TM, Kwok CL, Zhu T, Yip PS. Assessing suicide risk and emotional distress in Chinese social media: a text mining and machine learning study. J Med Internet Res. 2017;19(7):e243. [FREE Full text] [CrossRef] [Medline]
- de Choudhury M, Gamon M, Counts S, Horvitz E. Predicting depression via social media. Proc Int AAAI Conf Web Soc Media. 2021:128-137. [CrossRef]
- Fatima B, Amina M, Nachida R, Hamza H. A mixed deep learning based model to early detection of depression. J Web Eng. 2020:429-456. [CrossRef]
- Gupta B, Pokhriyal N, Gola KK. Detecting depression in Reddit posts using hybrid deep learning model LSTM-CNN. 2022. Presented at: 2022 2nd International Conference on Technological Advancements in Computational Sciences (ICTACS); October 10-12, 2022; Tashkent, Uzbekistan. [CrossRef]
- Jagtap N, Shukla H, Shinde V, Desai S, Kulkarni V. Use of ensemble machine learning to detect depression in social media posts. 2021. Presented at: 2021 Second International Conference on Electronics and Sustainable Communication Systems (ICESC); Coimbatore, India; August 4-6, 2021. [CrossRef]
- Wu J, Wu X, Hua Y, Lin S, Zheng Y, Yang J. Exploring social media for early detection of depression in COVID-19 patients. Proc ACM Web Conf. 2023:3968-3977. [CrossRef]
- Ríssola EA, Bahrainian SA, Crestani F. Anticipating depression based on online social media behaviour. 2019. Presented at: 13th International Conference on Flexible Query Answering Systems; July 2-5, 2019; Amantea, Italy.
- Ríssola EA, Bahrainian SA, Crestani F. A dataset for research on depression in social media. 2020. Presented at: UMAP '20: 28th ACM Conference on User Modeling, Adaptation and Personalization; July 14-17, 2020; Genoa, Italy. [CrossRef]
- Yohapriyaa M, Uma M. Multi-variant classification of depression severity using social media networks based on time stamp. In: Hemanth DJ, Pelusi D, Vuppalapati C, editors. Intelligent Data Communication Technologies and Internet of Things: Intelligent Data Communication Technologies and Internet of Things: Proceedings of ICICI 2021. Singapore. Springer; 2022.
- Wu MY, Shen C, Wang ET, Chen ALP. A deep architecture for depression detection using posting, behavior, and living environment data. J Intell Inf Syst. 2018;54(2):225-244. [CrossRef]
- Wongkoblap A, Vadillo MA, Curcin V. A multilevel predictive model for detecting social network users with depression. 2018. Presented at: 2018 IEEE International Conference on Healthcare Informatics (ICHI); June 4-7, 2018; New York, NY.
- Wang X, Zhang C, Ji Y, Sun L, Wu L, Bao Z. A depression detection model based on sentiment analysis in micro-blog social network. In: Li J, Cao L, Wang C, Tan KC, Liu B, Pei J, et al, editors. Trends and Applications in Knowledge Discovery and Data Mining. Heidelberg, Germany. Springer; 2013.
- Victor DB, Kawsher J, Labib MS, Latif S. Machine learning techniques for depression analysis on social media—case study on Bengali community. 2020. Presented at: 2020 4th International Conference on Electronics, Communication and Aerospace Technology (ICECA); November 5-7, 2020; Coimbatore, India.
- Tong L, Liu Z, Jiang Z, Zhou F, Chen L, Lyu J, et al. Cost-sensitive boosting pruning trees for depression detection on Twitter. IEEE Trans Affective Comput. 2022;14(3):1898-1911. [CrossRef]
- Tlachac ML, Rundensteiner E. Screening for depression with retrospectively harvested private versus public text. IEEE J Biomed Health Inform. 2020;24(11):3326-3332. [CrossRef] [Medline]
- Tejaswini V, Sathya Babu K, Sahoo B. Depression detection from social media text analysis using natural language processing techniques and hybrid deep learning model. ACM Trans Asian Low-Resour Lang Inf Process. 2024;23(1):1-20. [CrossRef]
- Sudhishna KS, Kumar CS, Kishore BS, Arun A. Comparative analysis of different machine learning algorithms to predict depression. 2023. Presented at: 2023 International Conference on Sustainable Computing and Smart Systems (ICSCSS); June 14-16, 2023; Coimbatore, India. [CrossRef]
- Stankevich M, Latyshev A, Kuminskaya E, Smirnov I, Grigoriev O. Depression detection from social media texts. 2019. Presented at: CEUR Workshop Proceedings; October 15-18, 2019; Kazan, Russia. URL: https://ceur-ws.org/Vol-2523/paper26.pdf
- Shekerbekova S, Yerekesheva M, Tukenova L, Turganbay K, Kozhamkulova Z, Omarov B. Applying machine learning to detect depression-related texts on social networks. In: Luhach AK, Jat DS, Bin Ghazali KH, Gao XZ, Lingras P, editors. Advanced Informatics for Computing Research. Singapore. Springer; 2021.
- Saini G, Yadav N, Kamath S S. Ensemble neural models for depressive tendency prediction based on social media activity of Twitter users. In: Rao UP, Patel SJ, Raj P, Visconti A, editors. Security, Privacy and Data Analytics: Select Proceedings of ISPDA 2021. Singapore. Springer; 2022.
- Ricard BJ, Marsch LA, Crosier B, Hassanpour S. Exploring the utility of community-generated social media content for detecting depression: an analytical study on Instagram. J Med Internet Res. 2018;20(12):e11817. [FREE Full text] [CrossRef] [Medline]
- Narynov S, Mukhtarkhanuly D, Omarov B, Kozhakhmet K, Omarov B. Machine learning approach to identifying depression related posts on social media. 2020. Presented at: 2020 20th International Conference on Control, Automation and Systems (ICCAS); Busan, South Korea; October 13-16, 2020. URL: https://www.researchgate.net/publication/347292707_Machine_Learning_Approach_to_Identifying_Depression_Related_Posts_on_Social_Media
- Mann P, Paes A, Matsushima EH. See and read: Detecting depression symptoms in higher education students using multimodal social media data. Proc Int AAAI Conf Web Soc Media. 2020;14:440-451. [FREE Full text] [CrossRef]
- Kumar SK, Dinesh N, Nitha L. Depression detection in Twitter tweets using machine learning classifiers. 2022. Presented at: 2022 Second International Conference on Interdisciplinary Cyber Physical Systems (ICPS); May 9-10, 2022; Chennai, India. [CrossRef]
- Kumar A, Pratihar V, Kumar S, Abhishek K. Predicting depression by analysing user tweets. In: Bajpai MK, Singh KK, Giakos G, editors. Machine Vision and Augmented Intelligence—Theory and Applications: Select Proceedings of MAI 2021. Singapore. Springer; 2021:633-644.
- Kour H, Gupta MK. Hybrid LSTM-TCN model for predicting depression using Twitter data. IEEE; 2022. Presented at: 2022 17th International Conference on Control, Automation, Robotics and Vision (ICARCV); December 11-13, 2022:167-172; Singapore. [CrossRef]
- Kour H, Gupta MK. Predicting the language of depression from multivariate twitter data using a feature‐rich hybrid deep learning model. Concurr Comput. 2022;34(24):e7224. [CrossRef]
- Kabir M, Ahmed T, Hasan MB, Laskar MTR, Joarder TK, Mahmud H, et al. DEPTWEET: A typology for social media texts to detect depression severities. Comput Hum Behav. 2023;139:107503. [CrossRef]
- Islam MR, Kamal ARM, Sultana N, Islam R, Moni MA, Ulhaq A. Detecting depression using k-nearest neighbors (KNN) classification technique. 2018. Presented at: 2018 International Conference on Computer, Communication, Chemical, Material and Electronic Engineering (IC4ME2); February 8-9, 2018:1-4; Rajshahi, Bangladesh. [CrossRef]
- Dey S, Nawshin AT, Hossain A, Rahman RM. Machine learning combined with PHQ-9 for analyzing depression in Bangladeshi metropolitan areas. 2022. Presented at: 2022 IEEE 11th International Conference on Intelligent Systems (IS); October 12-14, 2022:1-8; Warsaw, Poland. [CrossRef]
- Reece AG, Reagan AJ, Lix KLM, Dodds PS, Danforth CM, Langer EJ. Forecasting the onset and course of mental illness with Twitter data. Sci Rep. 2017;7(1):13006. [FREE Full text] [CrossRef] [Medline]
- Mariñelarena-Dondena L, Ferretti E, Maragoudakis M, Sapino M, Errecalde ML. Predicting depression: a comparative study of machine learning approaches based on language usage. Panam J Neuropsychol. 2017:42-54. [FREE Full text]
- Schwartz HA, Eichstaedt J, Kern ML, Park G, Sap M, Stillwell D, et al. Towards assessing changes in degree of depression through Facebook. In: Resnik P, Resnik R, Mitchell M, editors. Proceedings of the Workshop on Computational Linguistics and Clinical Psychology: From Linguistic Signal to Clinical Reality. Baltimore, MD. Association for Computational Linguistics; 2014:118-125.
- Mahnken K. Survey: More young people are depressed during the pandemic. The 74. 2017. URL: https://www.the74million.org/survey-more-young-people-are-depressed-during-the-pandemic-but-they-may-be-using-social-media-to-cope/ [accessed 2023-10-21]
- Ahmed A, Aziz S, Toro CT, Alzubaidi M, Irshaidat S, Serhan HA, et al. Machine learning models to detect anxiety and depression through social media: a scoping review. Comput Methods Programs Biomed Update. 2022;2:100066. [CrossRef] [Medline]
- Amedie J. The Impact of Social Media on Society [dissertation]. Santa Clara, CA. Santa Clara University; 2015. URL: http://scholarcommons.scu.edu/engl_176/2 [accessed 2025-02-25]
- Schwartz HA, Eichstaedt J, Kern M, Park G, Sap M, Stillwell D, et al. Towards assessing changes in degree of depression through Facebook. 2014. Presented at: Proceedings of the Workshop on Computational Linguistics and Clinical Psychology: From Linguistic Signal to Clinical Reality; February 22, 2025; Baltimore, MD. [CrossRef]
- Chandra Guntuku S, Preotiuc-Pietro D, Eichstaedt JC, Ungar LH. What Twitter Profile and Posted Images Reveal about Depression and Anxiety. In: ICWSM. 2019. Presented at: Proceedings of the International AAAI Conference on Web and Social Media; June 3–6, 2024:236-246; Buffalo, NY. URL: https://doi.org/10.1609/icwsm.v13i01.3225 [CrossRef]
- Wickramaratne PJ, Yangchen T, Lepow L, Patra BG, Glicksburg B, Talati A, et al. Social connectedness as a determinant of mental health: a scoping review. PLoS One. 2022;17(10):e0275004. [FREE Full text] [CrossRef] [Medline]
- Eichstaedt JC, Smith RJ, Merchant RM, Ungar LH, Crutchley P, Preoţiuc-Pietro D, et al. Facebook language predicts depression in medical records. Proc Natl Acad Sci U S A. 2018;115(44):11203-11208. [FREE Full text] [CrossRef] [Medline]
- Settanni M, Marengo D. Sharing feelings online: studying emotional well-being via automated text analysis of Facebook posts. Front Psychol. 2015;6:1045. [CrossRef] [Medline]
- Mendu S, Baglione A, Baee S, Wu C, Ng B, Shaked A, et al. A framework for understanding the relationship between social media discourse and mental health. Proc ACM Hum Comput Interact. 2020;4(CSCW2):1-23. [FREE Full text] [CrossRef]
- Benamara F, Moriceau V, Mothe J, Ramiandrisoa F, He Z. Automatic detection of depressive users in social media. 2018. Presented at: Conférence francophone en Recherche d'Information et Applications (CORIA); April 3-4, 2024; La Rochelle, France. URL: https://hal.science/hal-02942297/document
- Lachmar EM, Wittenborn AK, Bogen KW, McCauley HL. #MyDepressionLooksLike: Examining public discourse about depression on Twitter. JMIR Ment Health. 2017;4(4):e43. [FREE Full text] [CrossRef] [Medline]
- Rosen L, Whaling K, Rab S, Carrier L, Cheever N. Is Facebook creating “iDisorders”? The link between clinical symptoms of psychiatric disorders and technology use, attitudes and anxiety. Comput Hum Behav. 2013;29(3):1243-1254. [FREE Full text] [CrossRef]
- El Naqa I, Murphy MJ. What is machine learning? In: El Naqa I, Li R, Murphy MJ, editors. Machine Learning in Radiation Oncology: Theory and Applications. Cham, Switzerland. Springer International Publishing; 2015:3-11.
- Xu Y, Zhou Y, Sekula P, Ding L. Machine learning in construction: From shallow to deep learning. Dev Built Environ. 2021;6:100045. [FREE Full text] [CrossRef]
- Cannarile A, Dentamaro V, Galantucci S, Iannacone A, Impedovo D, Pirlo G. Comparing deep learning and shallow learning techniques for API calls malware prediction: a study. Appl Sci. 2022;12(3):1645. [FREE Full text] [CrossRef]
- Garg M, Saxena C, Saha S, Krishnan V, Joshi R, Mago V. CAMS: An annotated corpus for causal analysis of mental health issues in social media posts. ArXiv. Preprint posted online on July 11, 2022. 2022. [FREE Full text] [CrossRef]
- Salehinejad H, Sankar S, Barfett J, Colak E, Valaee S. Recent advances in recurrent neural networks. ArXiv. Preprint posted online on December 27, 2017. 2017. [FREE Full text]
- Sidey-Gibbons JAM, Sidey-Gibbons CJ. Machine learning in medicine: a practical introduction. BMC Med Res Methodol. 2019;19(1):64. [FREE Full text] [CrossRef] [Medline]
- Löwe B, Kroenke K, Herzog W, Gräfe K. Measuring depression outcome with a brief self-report instrument: sensitivity to change of the Patient Health Questionnaire (PHQ-9). J Affect Disord. 2004;81(1):61-66. [CrossRef] [Medline]
Abbreviations
BDI: Beck Depression Inventory |
CES-D: Center for Epidemiological Studies-Depression |
MOOSE: Meta-Analysis of Observational Studies in Epidemiology |
PHQ-9: Patient Health Questionnaire-9 |
Edited by T de Azevedo Cardoso; submitted 01.04.24; peer-reviewed by P Sivanandy, Y Hua, J Abbas, A Abdolmaleki; comments to author 19.06.24; revised version received 14.10.24; accepted 11.12.24; published 11.04.25.
Copyright©Doreen Phiri, Frank Makowa, Vivi Leona Amelia, Yohane Vincent Abero Phiri, Lindelwa Portia Dlamini, Min-Huey Chung. Originally published in the Journal of Medical Internet Research (https://www.jmir.org), 11.04.2025.
This is an open-access article distributed under the terms of the Creative Commons Attribution License (https://creativecommons.org/licenses/by/4.0/), which permits unrestricted use, distribution, and reproduction in any medium, provided the original work, first published in the Journal of Medical Internet Research (ISSN 1438-8871), is properly cited. The complete bibliographic information, a link to the original publication on https://www.jmir.org/, as well as this copyright and license information must be included.